Introduction
Developing therapeutics to cure diseases and improve human health is an overarching goal of biomedical research. The Commons supports the development of artificial intelligence (AI) and machine learning (ML) methods, with a strong bent towards developing the foundations of which ML methods are most suitable for drug discovery applications and why.
The Commons provides access to ML capability across therapeutic modalities and stages of discovery. It contains solvable ML tasks, ML-ready datasets, and curated benchmarks and leadearboards, all accessible via a Python package. Datasets in the Commons cover a wide range of therapeutic products (e.g., small molecules, biologics, gene editing therapies) across the entire discovery and development pipeline (e.g., target identification, hit discovery, lead optimization, manufacturing).
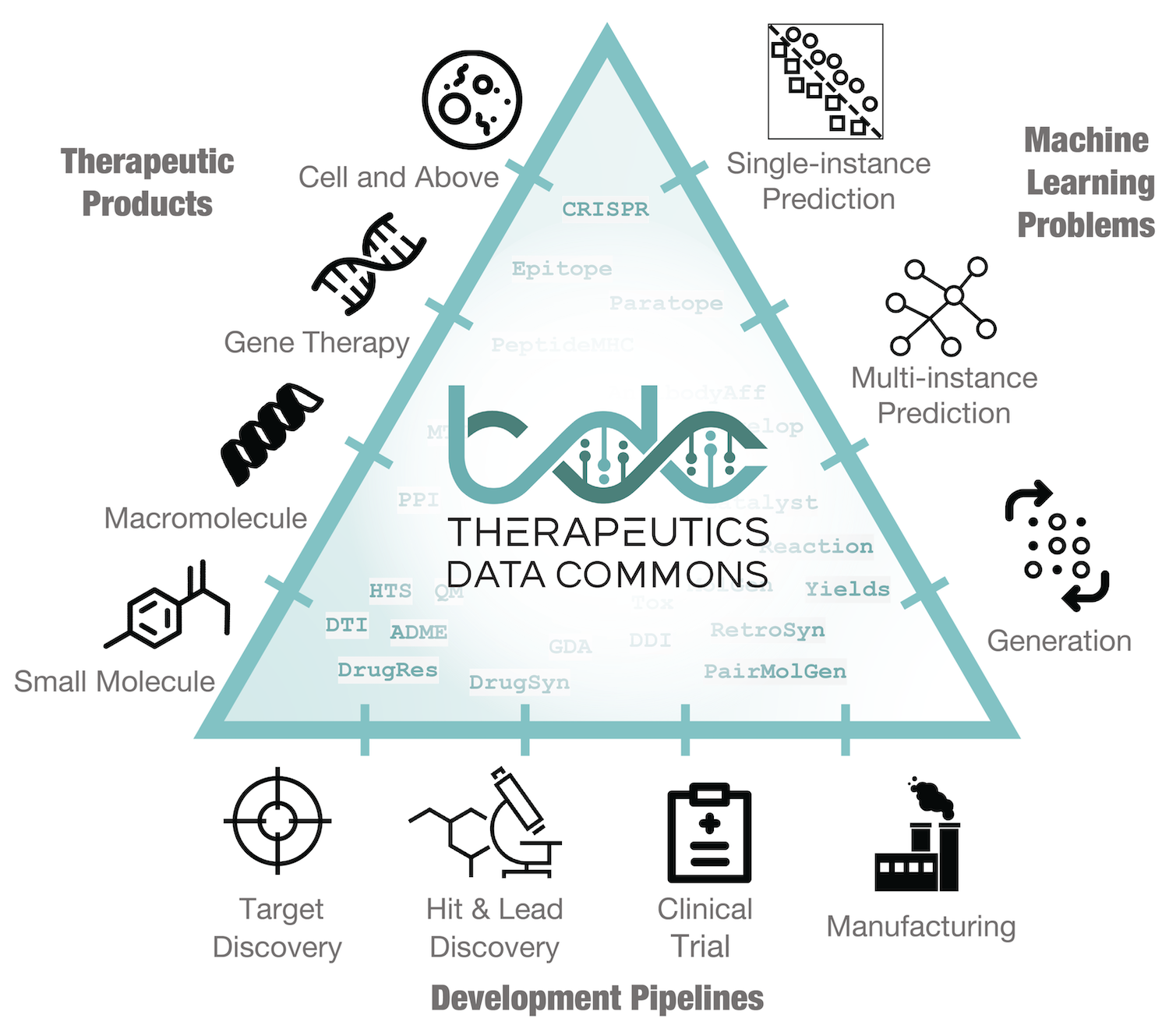
All datasets are ML-ready, meaning that input features are processed into an accessible format, such that scientists can use them directly as input to ML methods. TDC provides numerous functions for model evaluation, meaningful data splits, data processors, and oracles for molecule generation. All features of TDC are designed to easily integrate into any ML project, supporting the entire lifecycle of the project.
The Commons curates benchmarks for key therapeutic tasks. Every benchmark has a carefully designed ML task, ML-ready dataset, a public leaderboard, and a set of performance metrics to support model evaluation, providing effective indicators of the performance of ML methods in real-world scenarios.
The Commons lies at the nexus between artificial intelligence and drug discovery. Biologists and biochemists can pose ML tasks and identify relevant datasets that are carefully processed and integrated into Commons and formulated as scientifically valid ML tasks. ML scientists can rapidly obtain these tasks and develop ML methods to advance the therapeutic task past the state of the art and open up new opportunities.
Tiered Design of Therapeutics Data Commons: “Problem – ML Task – Dataset”
TDC has an unique three-tiered hierarchical structure. At the highest level, its resources are organized to support three types of problems. For each problem, we give a collection ML tasks. Finally, for each task, we provide a series of datasets.
The Commons outlines three major problems in the first tier:
- Single-instance prediction
single_pred
: Making predictions involving individual biomedical entities. - Multi-instance prediction
multi_pred
: Making predictions about multiple biomedical entities. - Generation
generation
: Generating new biomedical entities with desirable properties.
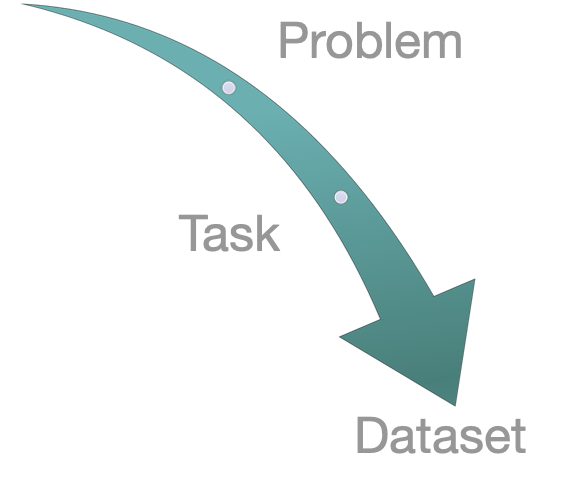
At the second tier, TDC is organized into ML tasks. Researchers across disciplines can use ML tasks for numerous applications, including identifying personalized combinatorial therapies, designing novel class of antibodies, improving disease diagnosis, and finding new cures for emerging diseases.
In the third tier, we provide multiple datasets for each task. For each dataset, we provide several splits of the dataset into training, validation, and test sets to evaluate model performance.
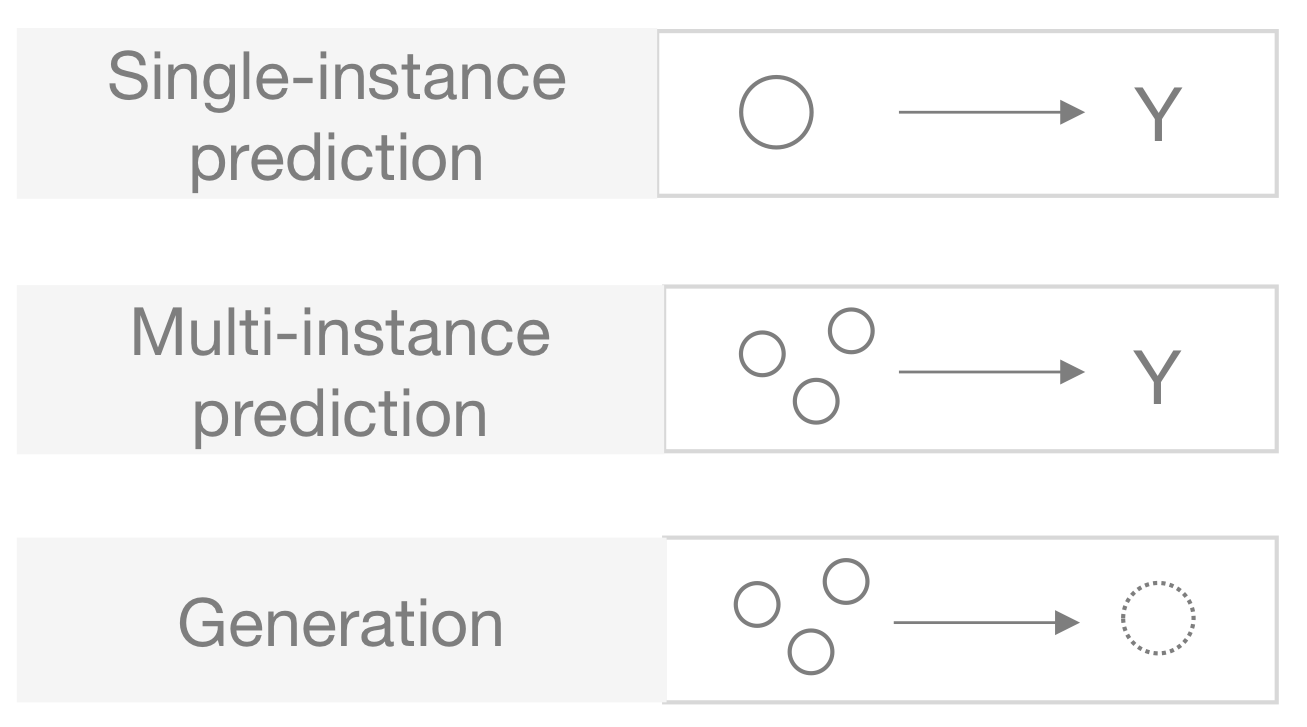
Installation
To install the TDC Python package, use the following:
pip install PyTDC
The installation of the package is hassle-free with minimum dependency on external packages.
Data Loaders
TDC provides intuitive, high-level APIs for both beginners and experts to create ML models in Python. Building off the modularized "Problem--ML Task--Dataset" structure, TDC provides a three-layer API to access any ML task and dataset.
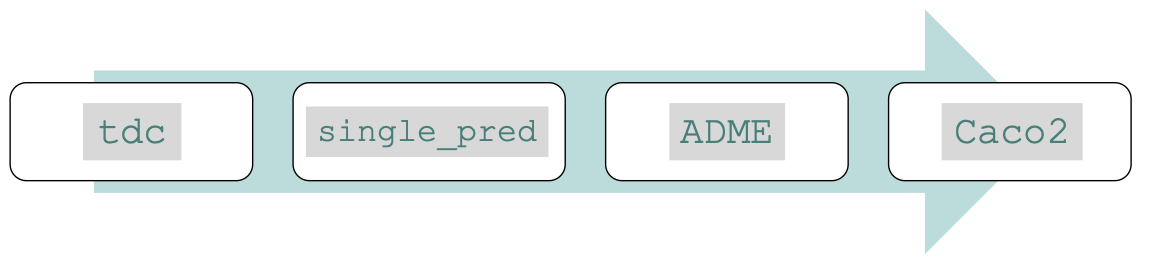
As an example, to obtain the Caco2
dataset from ADME
task in the single-instance prediction
problem do as follows:
from tdc.single_pred import ADME
data = ADME(name = 'Caco2_Wang')
df = data.get_data()
splits = data.get_split()
The variable df
is a Pandas object holding the entire dataset. By default, the variable splits
is a dictionary with keys train
, val
, and test
whose values are all Pandas DataFrames with Drug IDs, SMILES strings and labels. For detailed information about outputs, see Datasets documentation.
The user only needs to specify "Problem -- ML Task -- Dataset." TDC then automatically retrieves the processed ML-ready dataset from the TDC server and generates a data
object, exposing numerous data functions that can be directly applied to the dataset.
Ecosystem of Data Functions, Tools, Libraries, and Community Resources
TDC includes numerous data functions to support the development of novel ML methods and theory:
- Model Evaluation: TDC implements a series of metrics and performance functions to debug ML models, evaluate model performance for any task in TDC, and assess whether model predictions generalize to out-of-distribution datasets.
- Dataset Splits: Therapeutic applications require ML models to generalize to out-of-distribution samples. TDC implements various data splits to reflect realistic learning settings.
- Data Processing: As therapeutics ML covers a wide range of data modalities and requires numerous repetitive processing functions, TDC implements wrappers and useful data helpers for them.
- Molecule Generation Oracles: Molecular design tasks require oracle functions to measure the quality of generated entities. TDC implements over 17 molecule generation oracles, representing the most comprehensive colleciton of molecule oracles. Each oracle is tailored to measure the quality of AI-generated molecules in a specific dimension.
Public Leaderboards and Benchmarks
TDC provides leaderboards for systematic model evaluation and comparison. Every dataset in TDC can be thought of as a benchmark. For a model to be useful for a specific therapeutic question, it needs to consistently perform well across multiple datasets and tasks. For this reason, we group individual benchmarks into meaningful groups centered around therapeutic questions, which we refer to as benchmark groups. Dataset splits and evaluation metrics reflect real-world difficulty of therapeutic problems.
Explore Therapeutics Data Commons